Five Use Cases for AI/ML in Healthcare (Blog series – Part 1 of 3)
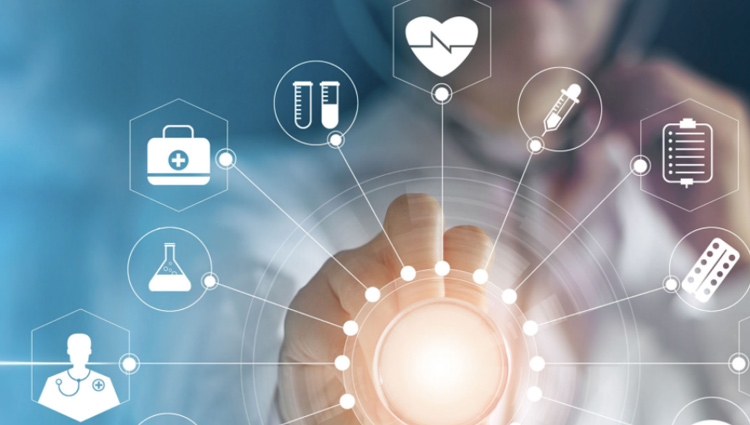
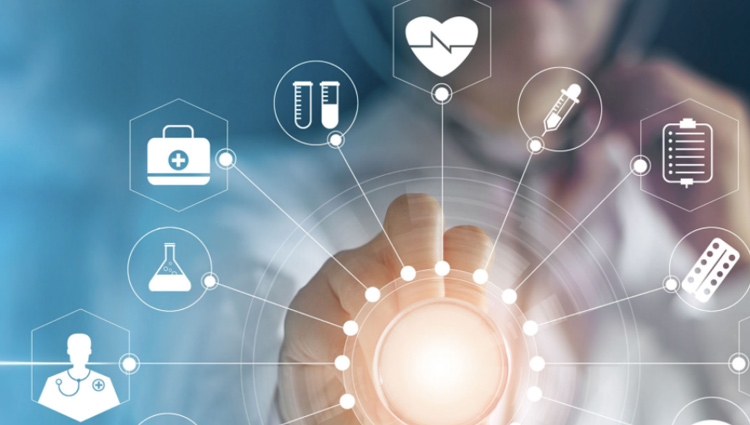
Technology has accelerated changes toward information-based healthcare delivery and management. Today’s multi-disciplinary approach to delivering better healthcare outcomes coupled with advanced imaging and genetic-based customized treatment models depend on AI/ML driven information systems.
At Robin.io, we believe machine learning is the life-saving technology that will transform healthcare. AI/ML challenges the traditional, reactive approach to healthcare. Of all the businesses, healthcare needs a predictive, proactive, and preventative approach.
In this three-part series, we will explore the promises of AI/ML on healthcare, the challenges faced by AI/ML projects, and finally how Robin CNP can help address some of these challenges.
The first part covers how AI/ML can help improve healthcare. Be sure to stay tuned for the next two parts of the series.
AI/ML in Healthcare
It goes no way unnoticed in the healthcare industry that AI/ML has suddenly gained a tremendous amount of interest. We are beginning to see AI/ML emerge in many of the software tools vendors offer to the industry, as well as through new products that have been customized to the healthcare setting.
Human resources shortage has become one of the greatest impediments for achieving universal health coverage globally. However, with artificial intelligence (AI), it can be changed. Data sources such as EHRs can be analyzed to generate prescriptions, detect disease states and provide proactive care.
A study by the Association of American Medical Colleges estimates that by 2025 there will be a shortfall of between 14,900 and 35,600 primary care physicians. That’s a problem because primary care physicians are the cornerstone of our health system. There are many factors behind this shortage, which is likely to be exacerbated by the aging of America as the massive baby boomer cohort enters its senior years. These factors include, among other things, lower reimbursement rates for primary care physicians in the U.S. and an ongoing primary care physician shortage in rural areas that have persisted for generations.
Artificial Intelligence and Machine Learning can help address this shortage. It can transform the practice of medicine by automating tasks and enhancing human decision-making.
It also has the potential to improve patient safety by alerting clinicians to patterns or signals they may have missed. We will explore five examples where AI/ML can help improve healthcare.
- Reducing readmissions
Reducing readmissions to hospitals is a significant challenge for healthcare providers. Medicare payments are linked to readmission rates, and failure to address this issue can be costly. Machine learning tools can have a transformative impact on care delivery, by providing clinicians with daily guidance as to which patients are most likely to be readmitted and how they might be able to reduce that risk. - Reduce Length-of-stay (LOS)
Length-of-stay (LOS) is the average number of days a patient spends in a hospital after being admitted and affects the quality of care, costs, and readmissions. Health systems can reduce LOS and improve other outcomes like patient satisfaction by identifying patients that are likely to have an increased LOS and then ensure that best practices are followed. - Predict Chronic Diseases
Chronic diseases (cancers, heart and lung diseases, mental health conditions, and others) are responsible for seven out of every 10 deaths in the United States each year. Predictive analytics has been identified as a key driver of healthcare cost and quality improvement. But hospital systems face technical, regulatory, and data integration hurdles in adopting predictive analytics. - Reduced ER Wait Times
With the growth of patient volumes and care complexity, managing the flow of patients in the ER has become more challenging for hospitals. The leaders turned to predictive analytics, new staffing models, and a system-wide change in culture to speed up care delivery for patients coming to the ER. - Gain Insights from Clinical notes
Clinical notes, hospital discharge summaries, reports, and other patient data are just one of the untapped areas for healthcare intelligence, but extracting that value is difficult. Health records are typically stored using a mix of data formats—including paper, email, and electronic health record systems—from different sources. Many times, the documentation and health records are not in the same place or even on the same device. Advanced NLP technologies have the potential to extract an enormous amount of value from clinical notes.
There is much to gain from using AI / ML in healthcare. It is relatively easy for healthcare organizations to realize the benefits of AI/ML. However, most fail to do so. Why? For one thing, it is due to various challenges concerning infrastructure, data governance, and tool provisioning. In the next part of the blog, we will talk about some of these challenges.