Four Key Challenges To Adopting AI/ML In Healthcare (Blog series – Part 2 of 3)
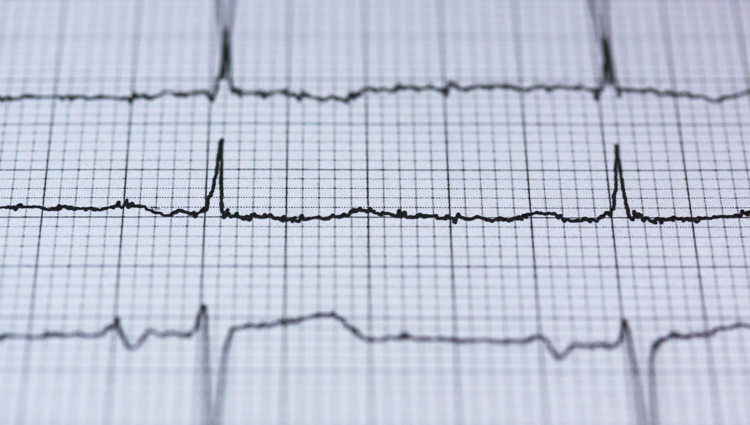
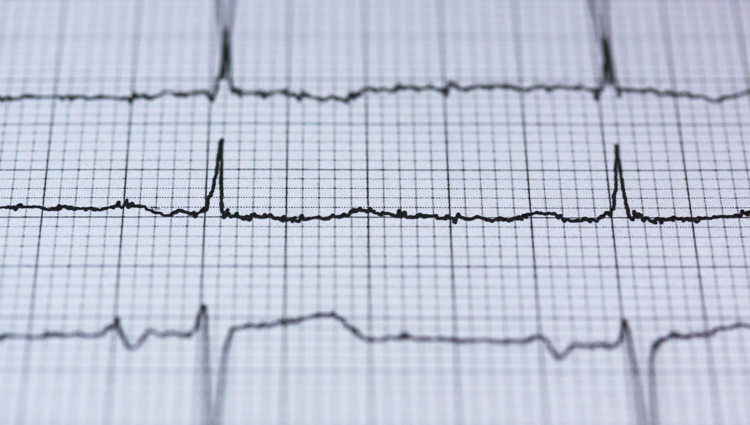
In part 1 of this series, we examined how AI/ML can help improve healthcare. AI/ML is an ambitious undertaking that promises to revolutionize healthcare. Getting excited is easy, but where do you start and why is it not just another empty promise? In fact, despite all these promises and futures, most AI/ML projects fail and don’t deliver. The failure rate of AI/ML projects is starting to make some wonder if this is real or hype. We at Robin believe that AI/ML projects can deliver successful outcomes by addressing some of the challenges commonly faced by many of the AI/ML projects. In this part, we will explore these challenges.
AI/ML Challenges
Per Gartner, about 80% of the AI/ML projects fail. That is a very high failure rate. Lack of data governance and the inflexibilities resulting from inadequate infrastructure and tooling are one of the major reasons for the failure of an AI/ML project.
AI/ML is not engineering and it is more experimental than engineering, so it requires more flexibility and agility in terms of infrastructure, platform, and tools. Projects that involve data-intensive applications are often delayed because of the complexity of deploying and managing these tools. This problem is made worse by the inflexibility of dynamically scaling compute, storage, and network infrastructure to meet Healthcare organization’s changing needs. This gets even more complicated when the enterprise deals with PII and other sensitive health data. The challenges become unreal when the ML engineers and data scientists require a self-service capability for their infrastructure.
To summarize, Healthcare organization have to solve the following challenges to enable their Data Science and AI/ML teams to unlock the value of their data faster to maintain competitive advantage:
- Time to provision the tools:
Most AI/ML projects fail because these tools are complex to set up, data sets are small and many cost prohibitive. Some of the tools take several weeks to provision and there is a constant need for more tools. Data scientists are often not happy or deliver results that would be better done by regular developers. The cloud providers are trying to help but for some new AI/ML workloads, they can’t convince most businesses especially the healthcare companies to run their code/ experiments on a public cloud. - Infrastructure – The infrastructure is often underutilized because it is dedicated to certain projects. It is not easy to move the workload around when they are directly deployed on the infrastructure. Prior one-off installations of products tend to be on highly divergent configurations, BOMs, hardware and software making each separate islands of operation, management and administration. This raises costs of gear, support, labor and more. Higher asset utilization, less floor space consumed, less power needed all lead to savings. Agility and time to market are improved immensely with a single platform to manage. This allows your staff to manage more with less enhancing productivity.
- Collaboration – As the whole process is experimental in nature, the AI/ML teams constantly require all stages of the experiments and not just the features set to be archived/snapshotted. The snapshots could be used to go back and recreate the experiment and sometimes to share and collaborate with other teams.
- Data Governance & Security – Data governance becomes difficult when the workflow involves environment provisioning and manual data copy. When your organization’s data governance model uses manual data copy, the business risks missing critical information and facing compliance issues. Self-service provisioning with proper audit trails can eliminate these risks. Thus, it is important for organizations with complex data to move to self-service tools.
This puts the AI/ML team and the platform/Infra team with the need to manage the data and platform much more efficiently.
In summary, the AI/ML team needs Quick provisioning of entire pipelines, self-manage day-two operations such as scaling and backups, the ability to take snapshots, collaborate across teams by sharing entire environments, Archive entire experiments.
On the other hand, the platform teams want to offer self-service, maintain visibility and control, enforce quota and consumption restrictions, improve hardware utilization, enforce separation of concerns and enforce data governance and security, and have a proper audit trail of all activities in the system.
Some challenges can be met by using public cloud solutions, but most healthcare organizations have privacy and regulatory concerns. Due to the sensitive nature of the health records, most healthcare organizations prefer on-prem solutions. But it is very difficult to build such systems from scratch without a heavy investment in time and effort. In the next part of the blog, we will show how Robin and Dell can help solve some of these challenges so healthcare organizations can realize the benefits of AI/ML.